Advanced neuroimaging and neurophysiology in Tuberous Sclerosis Complex
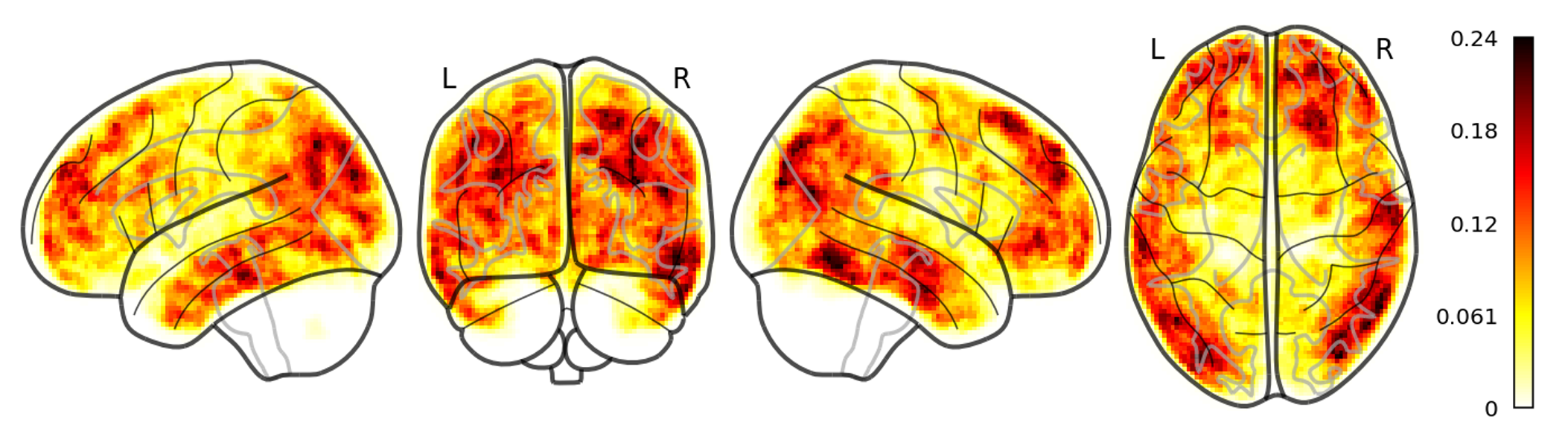
In neuroimaging, with my collaborators we were the first to describe decreased microstructural integrity in autism, intellectual disability, and severe epilepsy, allowing for the use of DTI as a biomarker for neurological outcome in TSC. Separately, we have demonstrated that tubers are poorly delineated, and that bother tubers and perituber tissue are dynamic, showing evolution over time. We have correlated abnormal white matter diffusion with neuropathology in TSC. A longitudinal imaging technique called Epilepsy Longitudinal Diffusion Imaging (ELoDI) is under development, aiming to visualize diffusion changes over time that are associated with epileptogenicity.
With EEG, functional connectivity measures, we found alterations of brain network properties common to both idiopathic and TSC-related autism, suggesting a common biological mechanism. Dr. Peters is responsible for EEG interpretation of all data collected in two large NIH-funded prospective multicenter trials of epilepsy in TSC, and in one industry sponsored trial of epilepsy in infants with TSC. Recently, we have published on increased EEG connectivity prior to spasms, and have applied lesion network mapping (LNM) to epileptic spasms in TSC, identifying two key hubs in a spasmogenic network.
Density Weighted Statistics (DWS) in Diffusion Tensor Imaging (DTI) tractography
In the Computational Radiology Laboratory, we proposed a novel solution for the problem of partial volume averaging in region-of-interest (ROI) analysis with DTI tractography. When voxels associated with a fiber tract are identified, the proportion of the voxel associated with the fiber tract is important. A common strategy to select a tract-based ROI has been to threshold the streamline density to identify voxels associated with a particular white matter tract (e.g., tract-based spatial statistics, TBSS). With such approaches, average DTI parameters of the region are then assessed by computing the mean value by summing the parameter over all the voxels above the threshold and dividing by the number of voxels in the region. However, partial volume effects confound the analysis. We describe the use of streamline density directly to enable an appropriate weighted average of diffusion tensor parameters. In our analysis, the diffusion tensor parameters of a region are calculated on the basis of equal weighting of each of the trajectories, rather than equal weighting of each voxel. This was published in a relatively small journal, but has been cited >100 times since 2012, as the novel method was adapted in the field.
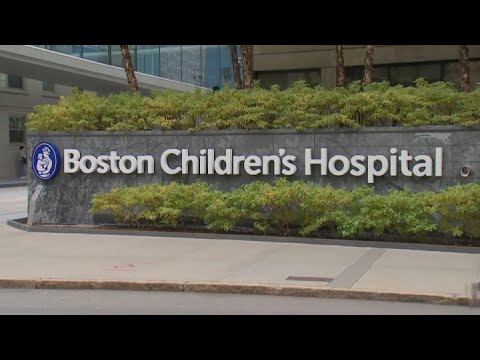
At the Computational Radiology Laboratory, we collaborated on a number of studies identifying dynamic patterns in scalp EEG of epileptic patients. We apply novel source localization techniques to these dynamics using the dynamic electrical source imaging (DESI) algorithm we developed. In these studies, Dr. Peters was responsible for collection, pre-processing and analysis of clinical routine and high density EEG data, and provide expertise to put the methods in appropriate clinical context of epilepsy surgery.
Dimensionality reduction with volumetric basis functions, and Lesion-Constrained Electrical Source Imaging
Scalp electroencephalography source localization is ill posed (there for all voltage patterns on the scalp, the solution is not unique because the number of candidate dipole sources vastly outnumbers the number of electrodes). Dimensionality reduction in the space of cortical sources is needed to improve computational and storage complexity.
First, to improve on classic volumetric methods which employ simplistic grid coarsening which ignore fine-scale anatomic structure, we introduced a novel approach to extend near-arbitrary spatial scaling to volumetric localization. Starting from a voxelwise brain parcellation, sub-parcels were identified from local cortical connectivity with an iterated graph cut approach. Spatial basis functions in each parcel were constructed using either a decomposition of the local leadfield matrix or spectral basis functions of local cortical connectivity graphs. We demonstrated, through use of both simulation and clinical data that our method improved reconstruction accuracy while reducing computational complexity.
Second, for application in multilesional epilepsy, we introduced a novel technique called lesion-constrained electrical source imaging (LC-ESI), based on a proposed head model in which the source solution is constrained to lesions. Using a goodness of fit analysis, we rank-ordered individual lesions by their ability to approximate interictal and ictal EEG data. Next, we color-coded these lesions to visualize the results in the patient’s own MRI, and compared to other available ESI techniques in epilepsy surgery clinical scenarios. This work was awarded the Cosimo Ajmone-Marsan Award for best original research paper in 2020 by the Journal of Clinical Neurophysiology, and supported by the American Clinical Neurophysiology Society.
Fully-automated model-based registration of high density EEG electrodes on patient-specific head models
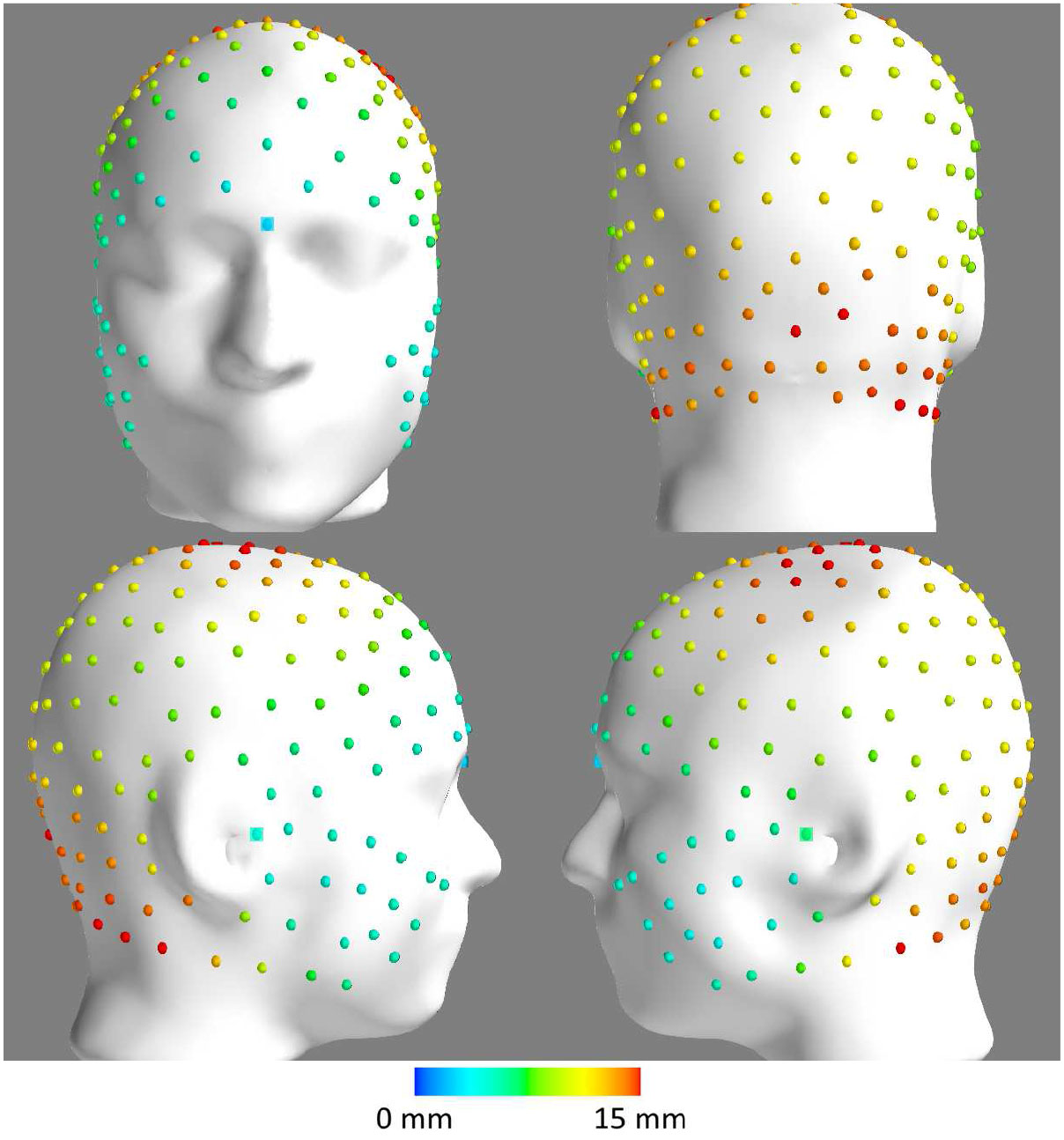
Electrical source imaging (ESI) is the most accurate when patient-specific head models are used, and the 3D positions of scalp electrodes are incorporated in the individualized bioelectric head model. Photogrammetry, the current gold standard, warrants cooperative patients and is resource intense, limiting application in a regular clinical workflow. We introduced a novel method of average electrode coordinates registered to the mean scalp mesh of a shape-constrained deformable head model used for tissue segmentation. Patient-specific electrode positions can be identified on the deformed scalp surface using pointbased correspondence after model adaptation. In simulations and with the use of clinical data, we demonstrated our method is accurate, more rapid, and eliminates any user dependent inaccuracy introduced in sensor registration – to ensure reproducible results. This work was selected as a featured article in the IEEE Transactions on Biomedical Engineering January Issue, 2021.
Machine learning and deep learning in rare disorders
In collaboration with the Computational Radiology Laboratory of Dr. Simon Warfield, we have applied machine learning and deep learning techniques to rare disease, which comes with its own unique challenges. Convolutional neural networks (CNNs) automatically detect patterns of interest in images and have demonstrated image-classification performance at or above the level of humans. These studies, however, require many thousands of images to train the CNNs – and therefore the study of rare disorders is precluded from this field. We have applied and refined techniques that allow for rare disorders to be studied with fully-connected convolutional neural networks. We have published on (1) data augmentation to increase the number of available training images, and on (2) improved learning from faulty image labeling (“noisy annotations”) in rare disorders. In ongoing work, we are also applying transfer learning (the use of pre-trained algorithms).
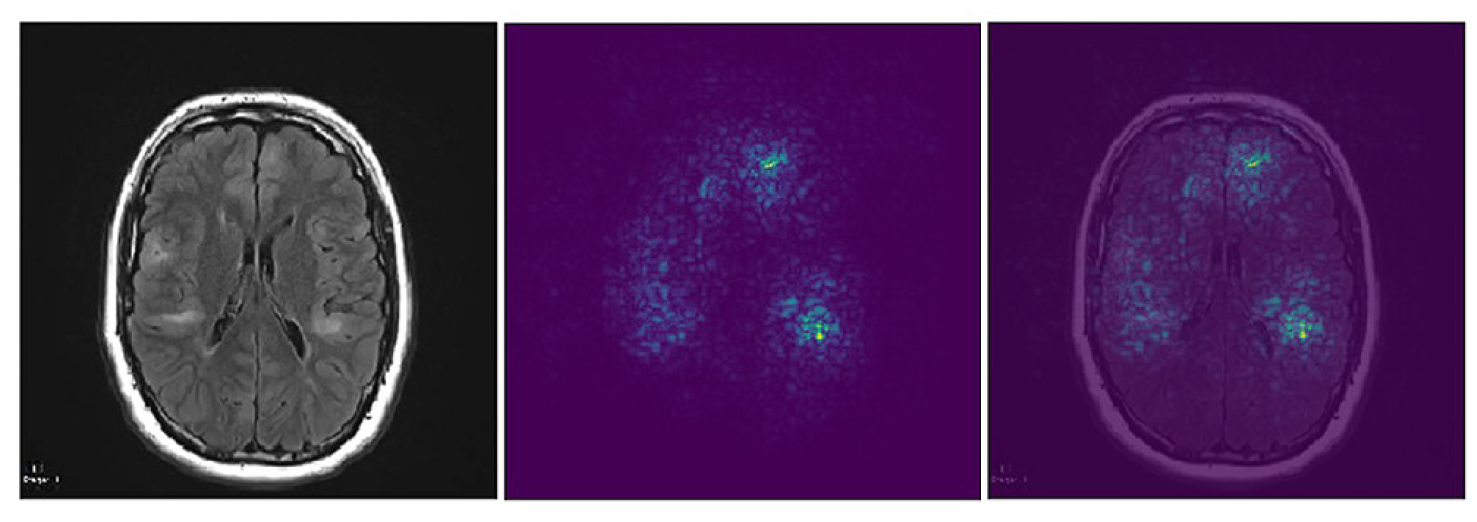